AI in Education Policy: Designing Pedagogy for an AI-Driven World
- James Purdy
- Apr 13
- 9 min read
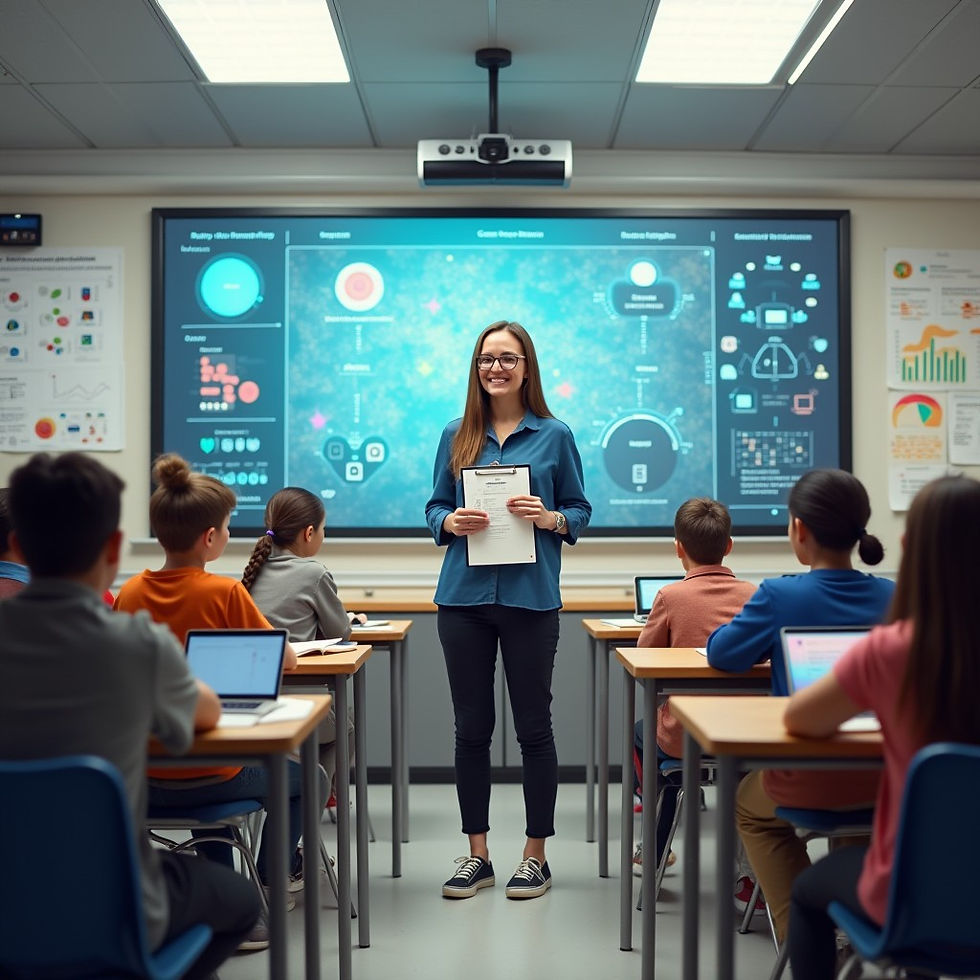
Key Takeaways
Post-secondary institutions will need to make AI competency training mandatory for workplace readiness.
I predict education needs a "hybrid AI pedagogy" approach balancing AI integration with AI-resistant pedagogies developing uniquely human capabilities.
Faculty need assessment frameworks that challenge students yet remain practical to evaluate.
[Affiliate disclosure: Your success fuels this operation. I have partnered with only the best AI companies who allow me to sell their fine services. If you can, please do me a solid and click around on these pictures a bit because some of these folks pay me for it. Think of it as a win-win: you get AI-powered resources that accelerate your growth, while supporting my amazing content.]
In my previous articles, I examined the AI policy governance gap and explored stakeholder perspectives. Today, I'm tackling what's missing in current approaches: reimagined pedagogy for an AI-integrated world.
The education sector faces unprecedented challenges. Only about 10–12% of North American school boards have formal AI policies, while 79% of educators report their districts lack clear guidance despite expecting increased AI use next year. Here, I propose a pedagogical framework addressing this gap, argue for mandatory AI competency training, and offer practical approaches balancing educational rigor with hands-on, implementable strategies.
The Post-Secondary AI Competency Gap
Higher education's most glaring oversight is treating AI as optional rather than core. While institutions debate permitting or prohibiting AI in assignments, workplaces increasingly expect AI proficiency.
A few forward-thinking institutions recognize this reality. Carnegie Mellon offers structured training in advanced prompting techniques while Michigan's Ross School of Business teaches "AI orchestration skills." These programs help students develop discipline-specific prompt libraries and evaluation frameworks.
Yet these remain exceptions. Most institutions either prohibit AI use or permit it without structured competency development. This creates a dangerous gap between education and workplace expectations.
Post-secondary institutions should make AI competency mandatory across all programs, focusing on:
Advanced prompting techniques for specific disciplines
Critical evaluation frameworks for AI outputs
Personalized AI systems augmenting domain expertise
Practical AI workflows for professional tasks
Hybrid AI Pedagogy
I propose a "hybrid AI pedagogy" – designing a single curriculum that maximizes the benefits of AI for students and teachers, while also creating space for learning experiences that cultivate human capacities AI cannot replicate.
This balanced strategy recognizes that while AI skills are essential, distinctly human capabilities remain the ultimate differentiator. I predict this will become critical in education, though few institutions currently think in these terms.
AI-Integrated Curriculum Design
Effective AI-integrated curriculum shouldn’t simply permit technology use but provide frameworks for thoughtful incorporation. The Ottawa Catholic School Board represents an early step, outlining AI applications for lesson planning, assessment, data analysis, and administrative tasks.
A truly effective approach must go beyond permission. It would provide structured guidance for curriculum redesign leveraging AI capabilities, creating assessment tasks with intentional AI use while emphasizing critical thinking, creativity, and learning process over final outputs.
AI-Resistant Pedagogical Frameworks
Simultaneously, institutions must develop learning experiences inherently resistant to AI replication – not merely to prevent academic dishonesty, but to cultivate uniquely human capacities. While most teachers have some experience with these alternative learning and teaching styles, they are usually relegated to a minor role in classroom practice and typically make up a small fraction of final grades. As AI becomes further entrenched in students’ lives, different and uniquely human skills will take on more significance.
This is the shift no one’s preparing for. Many education authorities remain focused on standardized, linear learning with easily measurable outcomes. As we’ve talked about before, AI is already quite good at teaching this kind of material as well as “gaming” these systems. I predict that non-linear learning processes and institutional metrics will need to be re-examined and given greater importance in the future if we want humans to remain competitive with AI.
Here are some of these learning models and possible assessment rubrics (mostly aimed at K–12):
Task-Based Learning
Beyond the theoretical framework, Task-Based Learning can be completed through carefully designed assessments that emphasize process documentation and iterative development beyond tangible outcomes. This could be anything from designing a product to making a new flag for the class to building a garden. The importance lies in giving students a grand task and allowing them to find their own way of completing it.
Example Assessment: "Design Evolution Portfolio"
Students create a software application, written piece, or design project through multiple documented iterations:
Initial concept development (documented through sketches, outlines, or pseudocode)
First draft or prototype with instructor feedback
Peer review session (students document feedback received and given)
Research and resources consultation (documented through annotated bibliography)
Revision based on feedback and research (with change tracking)
Final reflection analyzing how and why the project evolved
Assessment focuses on: Quality of iteration, responsiveness to feedback, depth of reflection, and improvement across versions rather than just the final product. Students submit all materials through a digital portfolio that showcases the evolution of their work. This multi-stage process with required human interactions is exceedingly difficult for AI to simulate.
Inquiry-Based Learning
The dynamic nature of authentic inquiry-based learning relies on real-time human interaction that current AI systems cannot replicate authentically.
Example Assessment for K–5: "Wonder Walk Investigations"
Elementary students explore scientific phenomena through guided outdoor inquiry:
Students take a "Wonder Walk" around the school grounds, recording things they're curious about on a simple observation sheet ("I wonder why...")
In small groups, students share their observations and select one wonder to investigate
Teacher facilitates a question-formulation technique where students refine their inquiry question
Students design a simple investigation method with teacher scaffolding
Groups collect data through observations, simple measurements, or photographs
Students create a visual display showing what they wondered, what they did, and what they discovered
Class "Wonder Conference" where students share findings and receive questions from peers
Assessment focuses on: Curiosity, question development, observational skills, and ability to explain thinking to others. Teachers use observational notes during the process and a simple rubric evaluating students' participation, question refinement, investigation design, and explanation ability. This approach harnesses children's natural curiosity while engaging them in physical space and real-time collaborative dialogue that AI cannot participate in authentically.
Place-Based Education
Learning rooted in specific local contexts creates experiences that even advanced AI models cannot authentically replicate.
Example Assessment for Grades 3–8: "Our Community Map Project"
Young students create multi-layered maps of their school neighborhood:
Class walking tour of the neighborhood, with students documenting observations through simple sketching and notes
Small groups each focus on different aspects (natural features, community helpers, historical sites, favorite places)
Students interview a family or community member about how the neighborhood has changed
Creation of physical map layers using different materials (tissue paper, fabric scraps, recycled items) representing different aspects of the community
Groups present their map layers and explain connections they discovered
Collective assembly of all layers into a 3D community map display
Students write personal reflections about what they value in their community and one way they could help improve it
Assessment focuses on: Observational skills, ability to represent physical space, making connections between different aspects of community, and personal engagement with place. Teachers assess through observation of participation, quality of physical map contributions, oral presentations, and written reflections. The combination of physical presence, tactile creation, and personal connection to specific local places makes this assessment impossible for AI to complete. Again, the focus is on the process rather than the product.
Embodied and Kinesthetic Learning
Physical engagement creates learning experiences that digital-only AI systems fundamentally cannot replicate.
Example Task: "Concept Choreography"
Students physically embody abstract concepts through movement and spatial relationships:
Research a complex system or process (photosynthesis, democratic governance, supply chains)
Design a movement sequence that represents the key components and relationships
Perform the sequence for peers, who must interpret the meaning
Refine based on peer interpretation and feedback
Create explanatory documentation connecting the movements to the concepts
Teach a portion of the sequence to others
Assessment focuses on: Accuracy of conceptual representation, creativity of expression, clarity of communication through movement, and ability to translate between kinesthetic and verbal/written modalities. This assessment requires bodily awareness and physical expression that AI systems simply cannot perform.
Service Learning
Authentic service learning depends on human connection, empathy, and adaptation to unpredictable real-world conditions.
Example Assessment: "Intergenerational Knowledge Exchange"
Students partner with senior community members on a project addressing community needs:
Initial community listening sessions to identify senior priorities and concerns
Development of project proposals addressing identified needs
Weekly collaboration sessions with senior partners
Adaptation of project based on emerging challenges and opportunities
Implementation of small-scale intervention or solution
Joint reflection with senior partners on outcomes and learning
Assessment focuses on: Quality of relationship building, responsiveness to partner needs, adaptability to changing circumstances, and mutual learning documented by both student and community partner. The unpredictable human interactions and emotional intelligence required make this form of assessment impossible for AI to simulate.
Making It Work: Practical Implementation
1. Ready-to-Adapt Assessment Templates
Institutions need assessment frameworks designed for both AI integration and resistance, including:
Elements suitable for AI personalization
Structured prompts for generating variations
AI-resistant components maintaining pedagogical integrity
Efficient evaluation rubrics focused on process over product
2. Professional Development for Hybrid AI Pedagogy
As we discussed in the last article, educators need specialized training in both AI integration and AI-resistant pedagogy. The "EThICAL" framework provides a systematic approach:
Excite: engage staff with AI possibilities
Tailor and Hone: identify specific challenges AI can address
Identify: determine available data and its relevance
Collect: gather additional data needed
Apply: select and implement appropriate AI techniques
Learn: analyze results and learn from the data
Iterate: refine the process based on outcomes
3. Time and Implementation Support
Teachers need dedicated time for training, experimentation, and adaptation. Without this support, even the best policies will fail. The EdWeek Research Center found 78% of educators lack the bandwidth to address AI alongside existing responsibilities.
A Way Forward: Subject-Specific Integration
AI policy must acknowledge that implementation varies across disciplines. Lauren Bialystok, professor at the University of Toronto, notes: "What may be admissible for using AI in a science class may be less admissible in an English class, or vice versa."
Departments also need to develop discipline-specific guidance which can maximize both the critical human elements as well as leveraging the exciting possibilities of AI in order to meet objectives.
STEM disciplines: Enhance problem-solving while preserving conceptual understanding
Humanities: Support research and ideation while maintaining human-centered analysis
Arts: Leverage AI for technical aspects while preserving creative vision
Professional programs: Simulate workplace AI integration while developing critical evaluation skills
This maintains coherent ethical principles while accommodating diverse disciplinary impacts.
Looking Ahead: The Imperative for Action
Educational institutions that fail to develop comprehensive AI policies risk producing graduates unprepared for an AI-integrated future. Those prohibiting AI create artificial environments disconnected from workplace realities, while those permitting AI without structured competency development produce graduates lacking sophisticated skills.
A hybrid AI pedagogy approach offers a middle path developing both technical capabilities and distinctly human skills. It’s time schools start designing for what AI can’t do.
By making AI competency core while preserving spaces for uniquely human learning, institutions can prepare students for a future of human-AI collaboration.
In our next article, I'll explore administrative challenges of implementing comprehensive AI policies, examining resource implications, governance structures, and change management approaches.
This is the third article in our "AI in Education" series (3/6). If you missed them, check out our first article examining AI policy's current state and our second article exploring student, teacher and administrator perspectives on AI policy. Want to discuss AI policy development for your institution? Reach out—we're stronger when we learn from each other.
References
Barrett, A., & Pack, A. (2023). Not Quite Eye to A.I.: Student and Teacher Perspectives on the Use of Generative Artificial Intelligence in the Writing Process. International Journal of Educational Technology in Higher Education, 20(1), 59.
Chan, C.K.Y., & Hu, W. (2023). Students' Voices on Generative AI: Perceptions, Benefits, and Challenges in Higher Education. International Journal of Educational Technology in Higher Education, 20(1), 43.
Dusseault, B., & Lee, J. (2023, October). AI is Already Disrupting Education, but Only 13 States are Offering Guidance for Schools. Center on Reinventing Public Education.
Klein, A. (2024, February 19). Schools Are Taking Too Long to Craft AI Policy. Why That's a Problem. Education Week.
Luckin, R., Cukurova, M., Kent, C., du Boulay, B. (2022). Empowering Educators to Be AI-Ready. Computers and Education: Artificial Intelligence, 3, 100076.
Moorhouse, B.L., Yeo, M.A., & Wan, Y. (2023). Generative AI Tools and Assessment: Guidelines of the World's Top-Ranking Universities. Computers & Education Open, 5, 100151.
Ottawa Catholic School Board. (2024). Artificial Intelligence at the OCSB.
Perkins, M., Furze, L., Roe, J., & MacVaugh, J. (2024). The Artificial Intelligence Assessment Scale (AIAS): A Framework for Ethical Integration of Generative AI in Educational Assessment. Journal of University Teaching and Learning Practice, 21(06).
Puzic, S. (2023, September 2). School boards grappling with AI use in classroom, but formal policies still elusive. Lethbridge News Now.
Quality Assurance Agency for Higher Education. (2023). Reconsidering Assessment for the ChatGPT Era.
Comments